Projects
Artificial intelligence enabled electrocardiography system
Participants
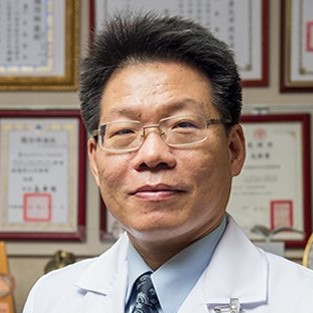
Shih-Hua Lin
Adviser
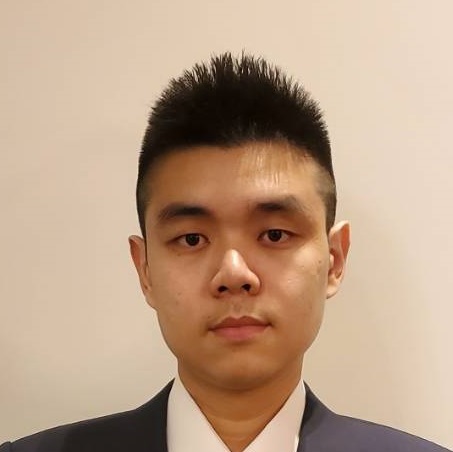
Chin Lin
Technical PI
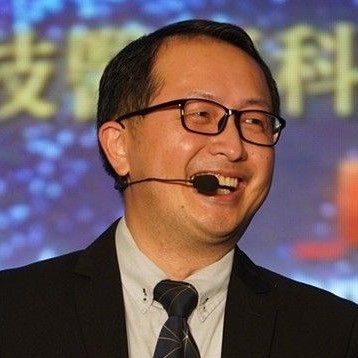
Chin-Sheng Lin
Clinical PI
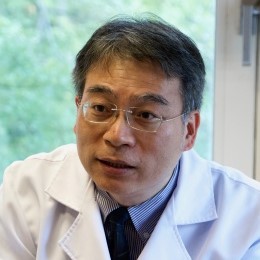
Wen-Hui Fang
Community PI
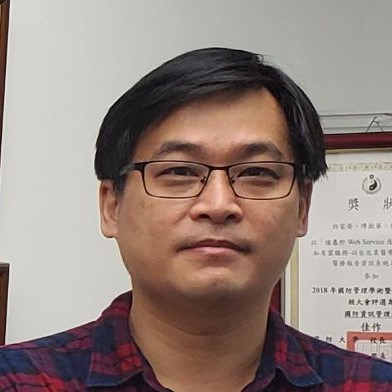
Chia-Jung Hsu
Engineer
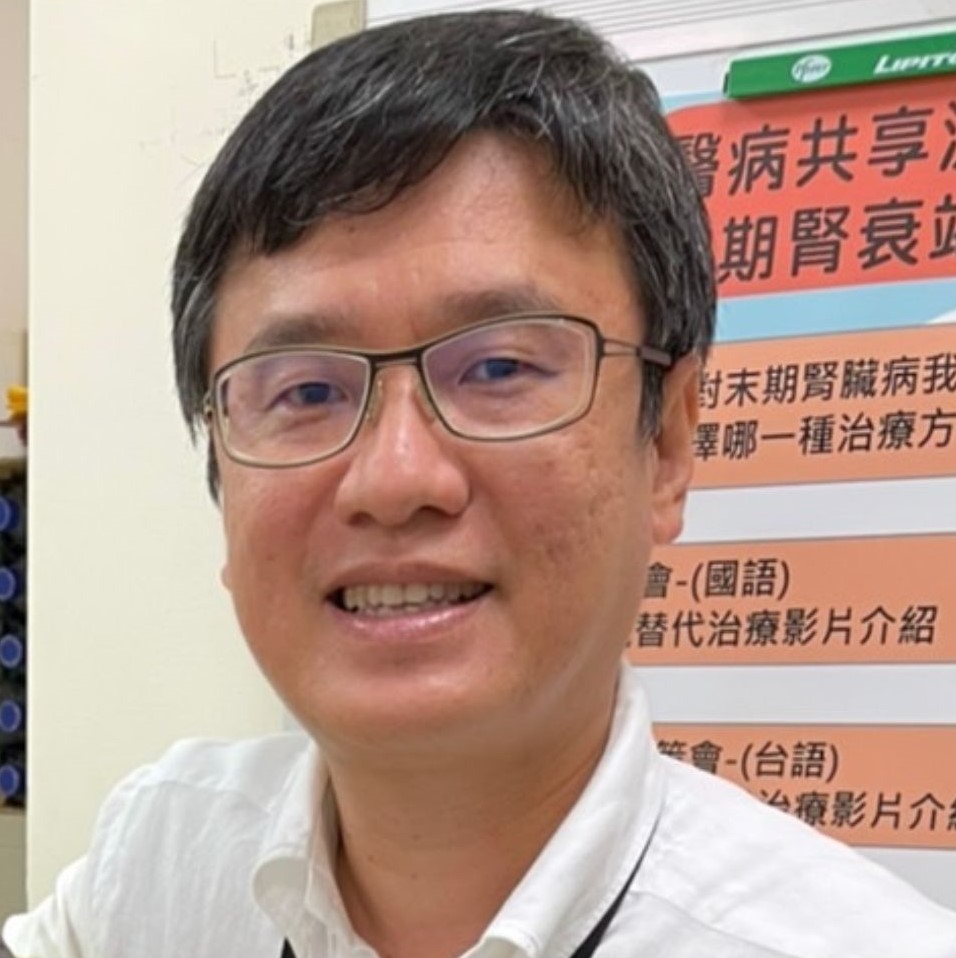
Yu-Juei Hsu
Clinical PI
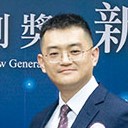
Shi-Hung Tsai
Emergency PI
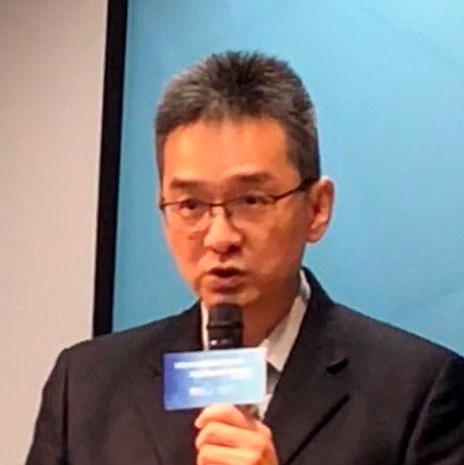
Chia-Cheng Lee
IT manager
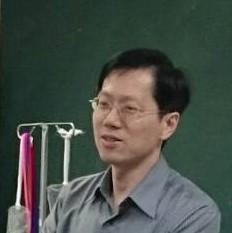
Sy-Jou Chen
Emergency physician
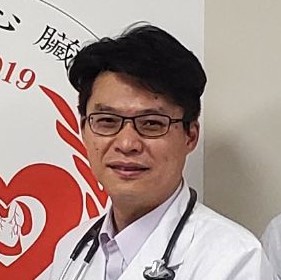
Wen-Yu Lin
Cardiologist
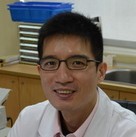
Ban-Yen Liu
Cardiologist
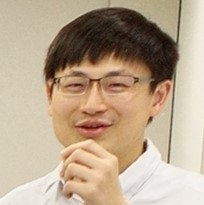
Wen-Cheng Liu
Cardiologist
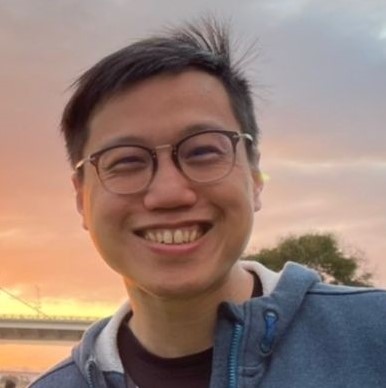
Da-Wei Chang
Cardiologist
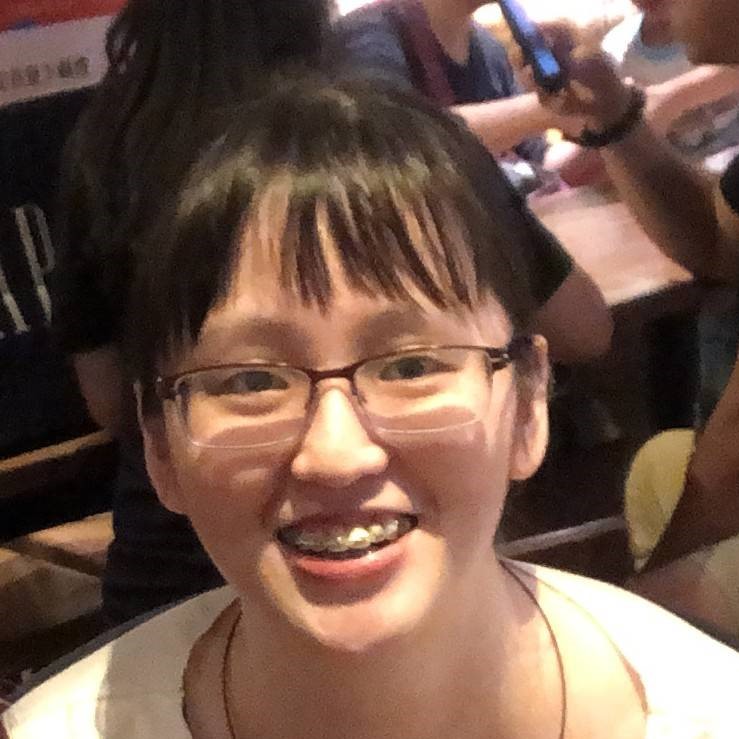
Yu-Lan Liu
Cardiologist
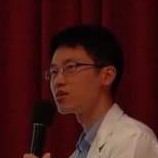
Ping-Huang Tsai
Nephrologist
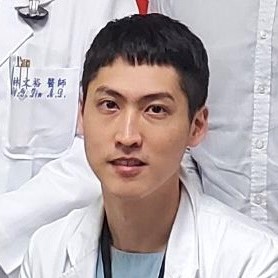
Chiao-Hsiang Chang
Cardiologist
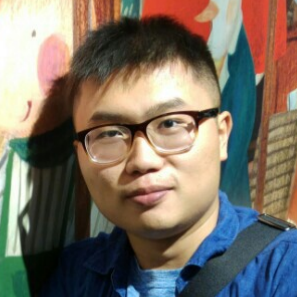
Hung-Yi Chen
Cardiologist
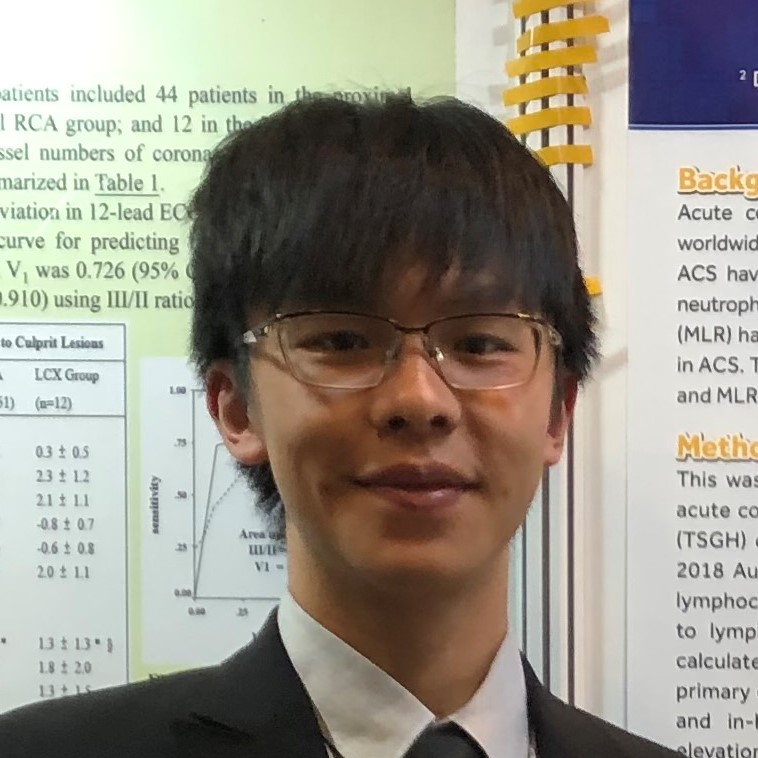
Chiao-Chin Lee
Cardiologist
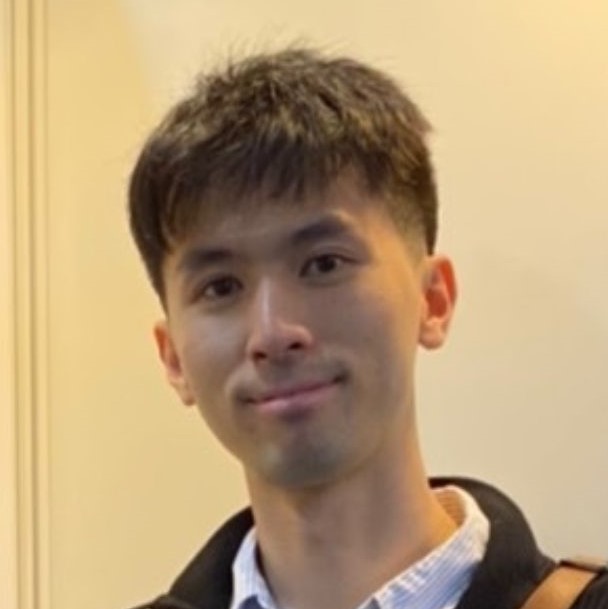
Wei-Ting Liu
Cardiologist
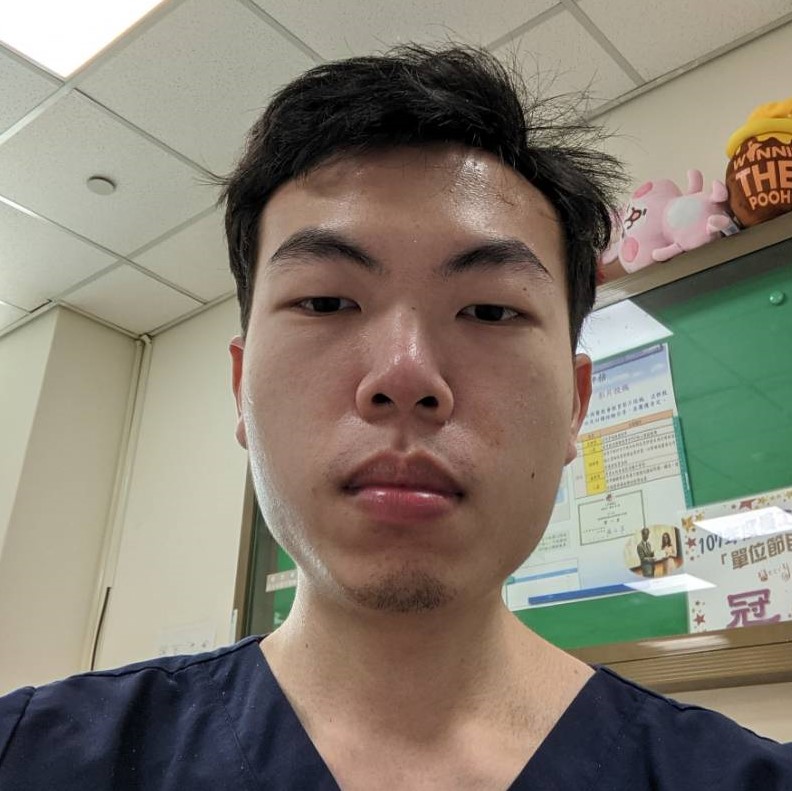
Yu-Cheng Chen
Cardiologist
Summary
1Nat Med, 30(5):1461-1470.
2NEJM AI, 1(7):AIoa2400190.
3npj Digital Medicine, 5(1):8.
4EuroIntervention, 17(9):765-773.
5Can J Cardiol, 38(2):160-168.
6Can J Cardiol, 40(7):1310-1321.
7Commun Med, 4(1):42.
8Clin Chim Acta, 536:126-134.
9J Med Syst, 47(1):81.
10J Med Syst, 48(1):67.
11JMIR Med Inform, 8(3):e15931.
12J Pers Med, 11(11):1149.
13J Pers Med, 12(7):1150.
14Eur J Trauma Emerg Surg, 48(4):3317-3326.
15Int J Environ Res Public Health, 18(7), 3839.
16J Endocr Soc, 5(9):bvab120.
17Aging, 16(10):8717-8731.
18J Pers Med, 12(3):455.
19Front Med, 9:870523.
20J Pers Med, 11(8):725.
21Front Cardiovasc Med, 9:895201.
22J Pers Med, 12(2):315.
23Digital Health, 9:20552076231187247.
24Front Cardiovasc Med, 9:754909.
25Diagnostics, 13:2723.
26Acta Cardiol Sin, 39(6):913–928.
27Am J Crit Care, 34(1):41-51.
28We shared our works to the Discovery.
Algorithm development
Participants
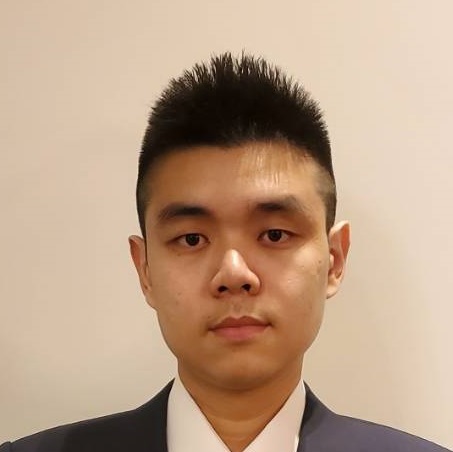
Chin Lin
Adviser
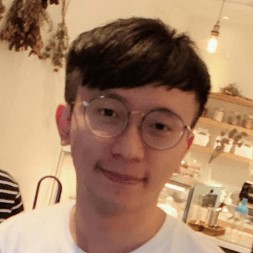
Yu-Sheng Luo
Post doct
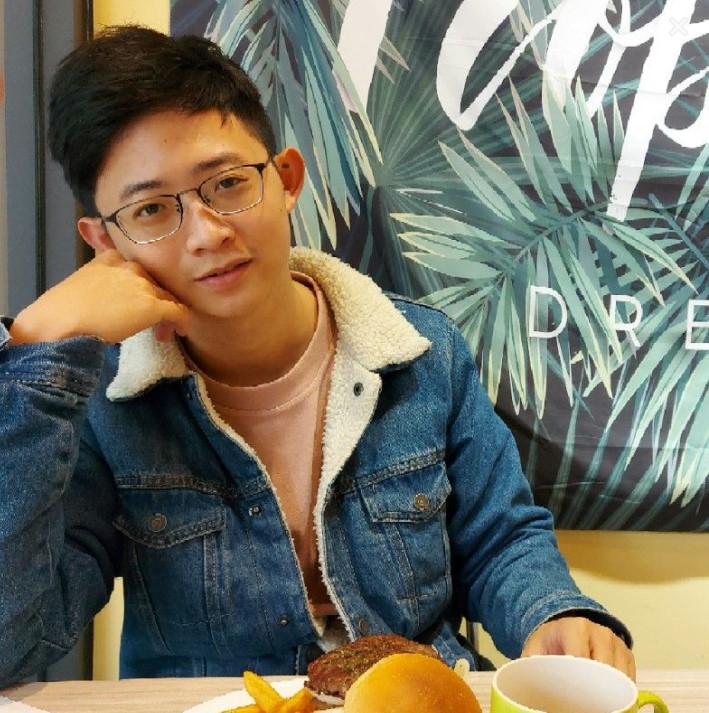
Chun-Ho Lee
Master student
Summary
Deep learning is a data-driven algorithm, so it is necessary to understand the limitations of medical data and design suitable algorithms to build an accurate medical artificial intelligence system. We consider that main differences between medical field and other fields and solutions are as follows:
1. Unsupervised learning aided supervised learning to solve the rare data problem: Because the medical data involves manual operations and expensive instruments, the amount of labeled data is scarce compared with other industries. Moreover, the incidence and prevalence of more fatel diseases is lower compared with other common diseases. We need to face this important limitation rather than selecting sufficient data for research. Transfer learning is a common solution in deep learning training that can effectively reduce the number of samples required. Our main work is to use a large amount of unlabeled data for unsupervised algorithms, and apply them to supervised learning.1
2. Combination of multi-level statistical models and deep learning to create personalized artificial intelligence: The response of each indivial to the same treatment is different, so it is impossible to use one artificial intelligence models for accurately appling in everyone. Therefore, it is very important to establish a personalized artificial intelligence model, but it is difficult to train each person's data separately in medical field. The multi-level data analysis in statistics shows how to use the distribution assumptions to construct personalized predictions. Even if only one record also can be used to construct a personalized model, our research attempts to apply this concept to deep learning training. The new age medical artificial intelligence can dimamicly revise the prediction results.2
3. To design special prediction functions and loss functions: When using artificial intelligence models in the medical field, interpretability and confidence issues often arise. For example, if the data quality is poor, it may be advisable for frontline operators to perform the check again immediately. This is particularly important in prospective trials.3
4. Special accuracy evaluation metric: Medical artificial intelligence research often ignores the problem of variable dependence in medical data, such as the relationship between electrocardiograms and age and gender, and the relationship between gender and age and bone density, leading to confusion about whether the relationship between electrocardiograms and bone density is based on a real causal relationship. Because of the powerful feature extraction ability of deep learning models, AI currently often learns false correlations to establish predictive logic, which will reduce the accuracy of external validation in the future, so it is necessary to design appropriate evaluation indicators for this problem.4
1Comput Methods Programs Biomed, 231:107359.
2Eur Heart J-DH, ztac072.
3Digital Health, 8:20552076221143249.
4Unpublished data.
Medical image analysis
Participants
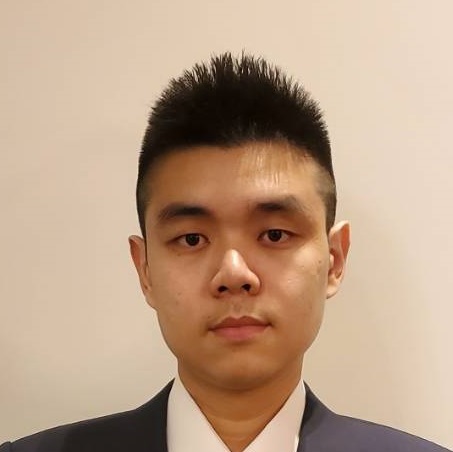
Chin Lin
Adviser
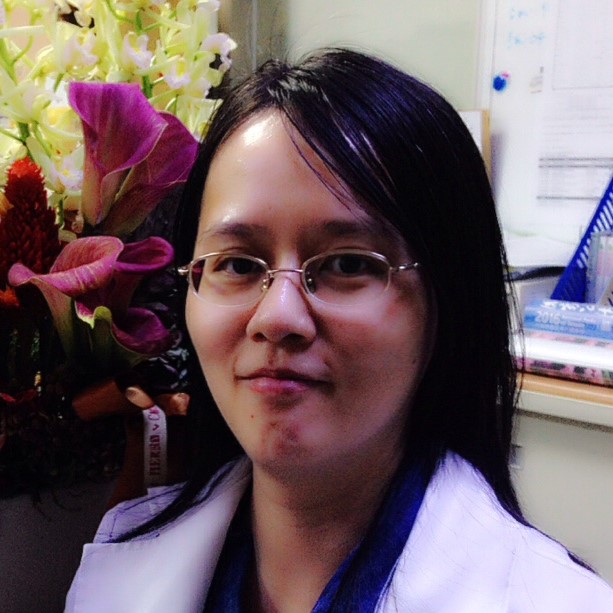
Yi-Ying Wu
Hematologist
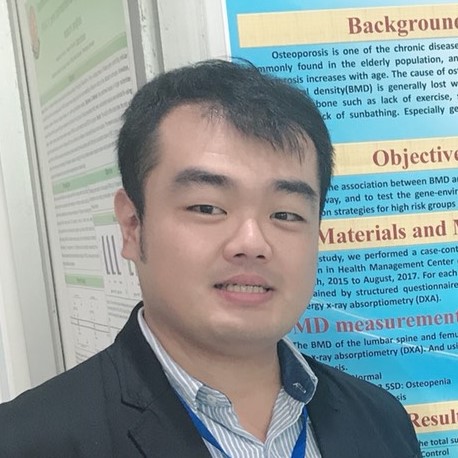
Dung-Jang Tsai
Research fellow
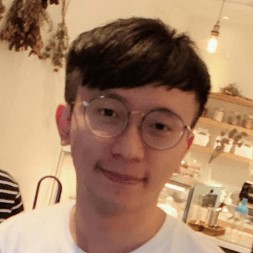
Yu-Sheng Luo
PhD student
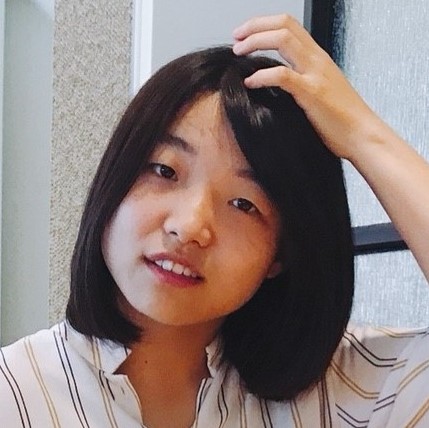
Ying-Chu Chen
MS student
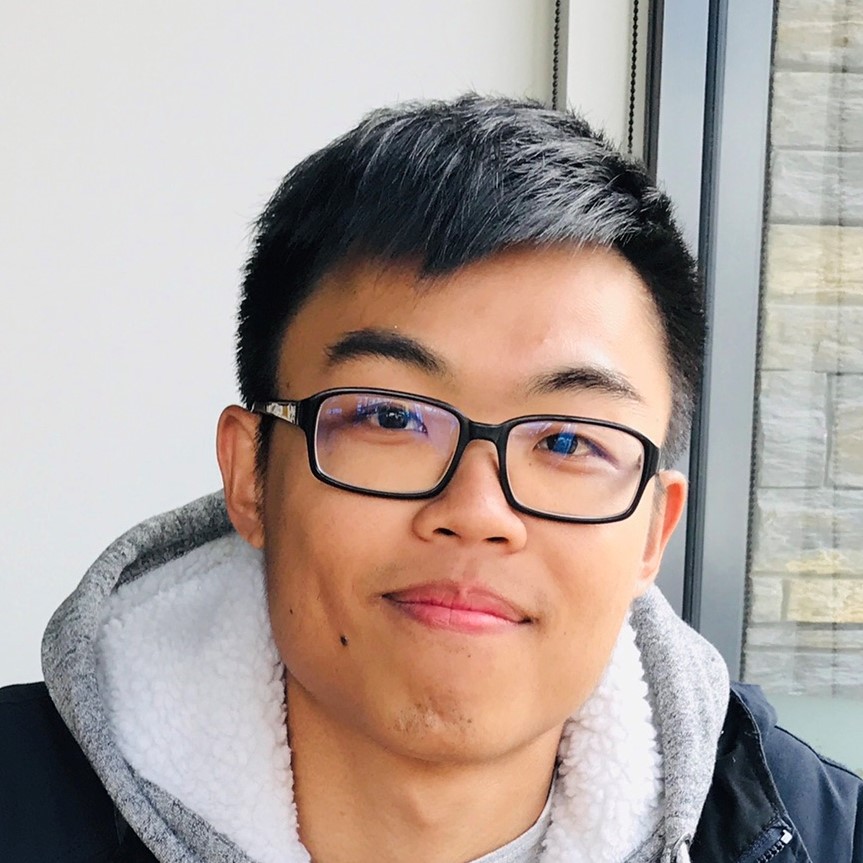
Xin-An Lin
Engineer
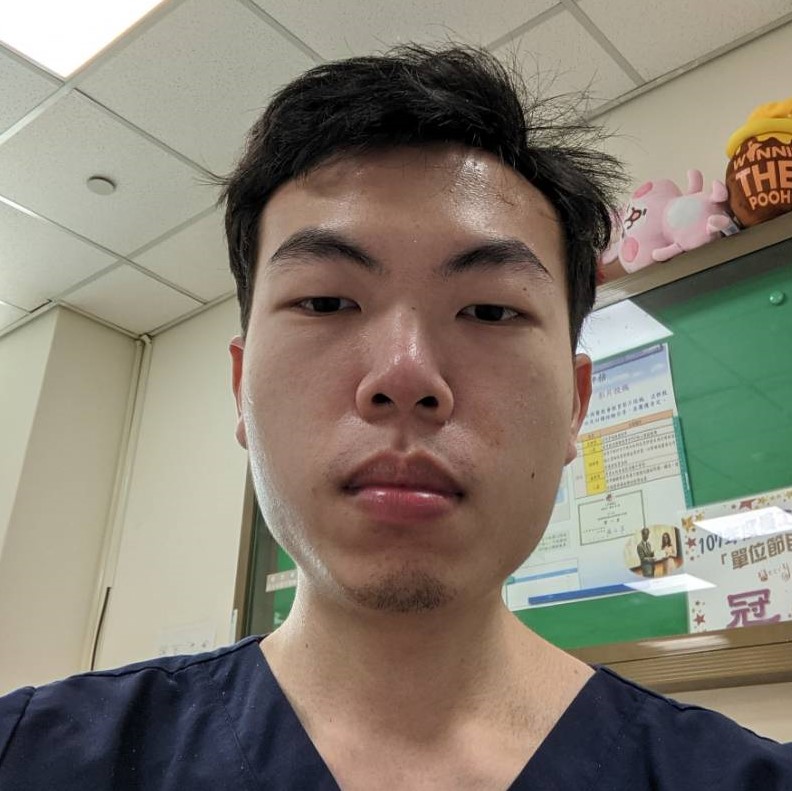
Yu-Cheng Chen
Cardiologist
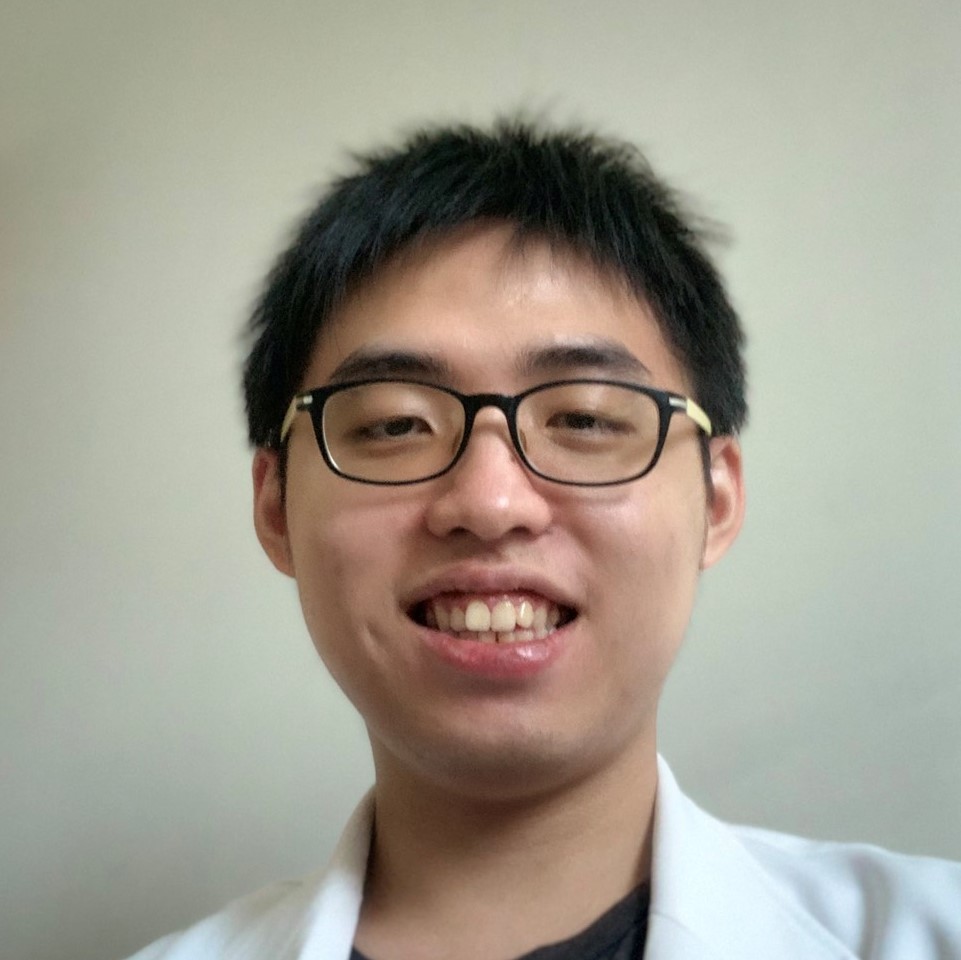
Hao-Chun Liao
BS student
Summary
Reference:
1Nat Med, 27(10):1735-1743.
2Radiology, 311(3):e231937.
3Can J Cardiol, 38(6):763-773.
8Can J Cardiol, 38(2):160-168.
5J Med Syst, 47(1):81.
6J Med Syst, 48(1):12.
7JMIR Med Inform, 8(4):e15963.
8Digital Health, 9:20552076231191055.
Medical Natural Language Processing
Participants
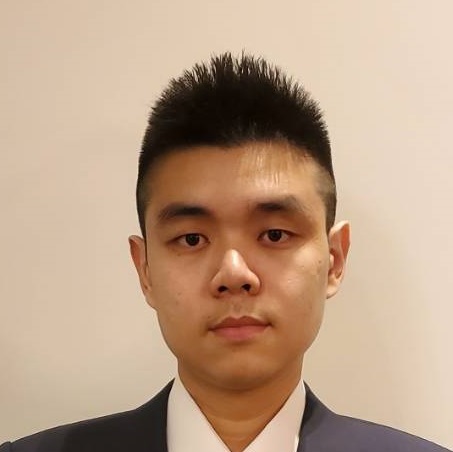
Chin Lin
Adviser
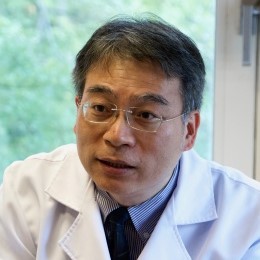
Wen-Hui Fang
Clinical expert
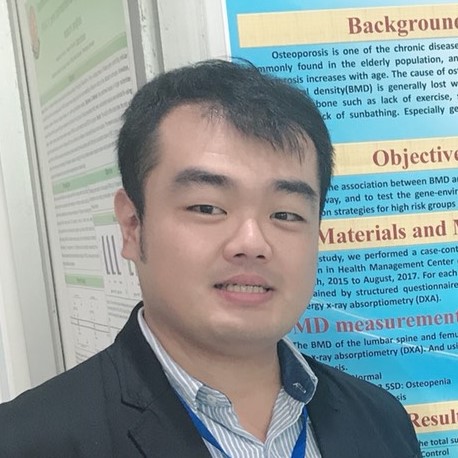
Dung-Jang Tsai
Research fellow
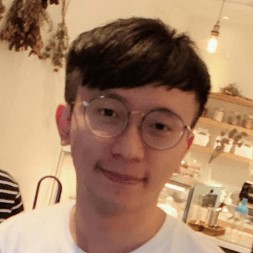
Yu-Sheng Luo
PhD student
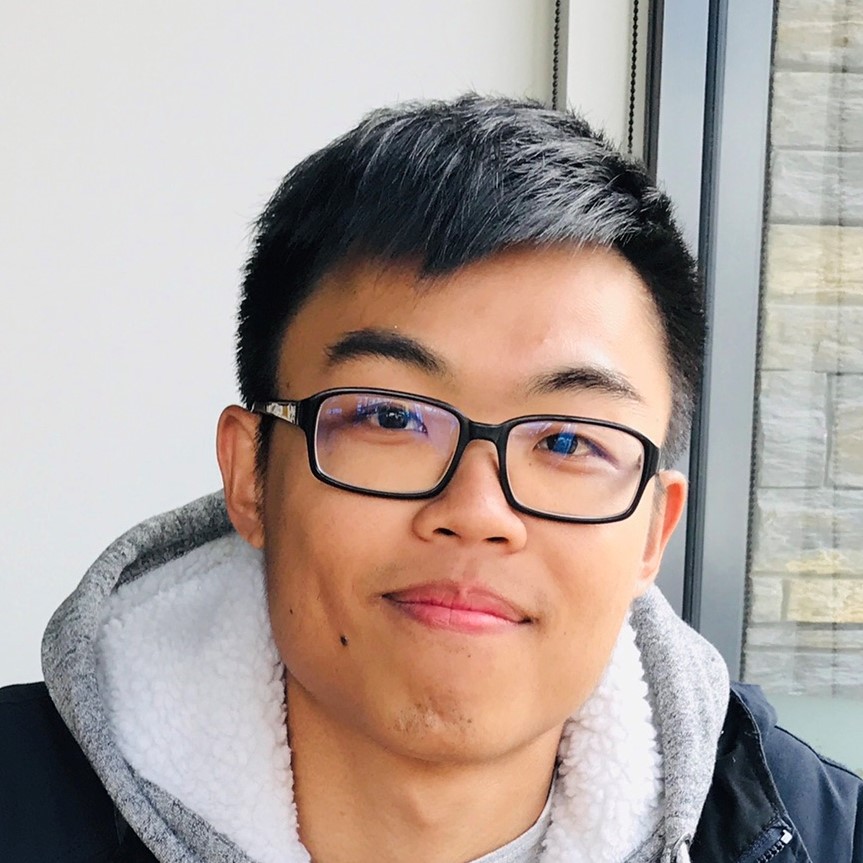
Xin-An Lin
Engineer
Summary
Reference:
1J Med Internet Res, 19(11):e380.
2JMIR Med Inform, 7(3):e14499.
3Healthcare, 9(10):1298.